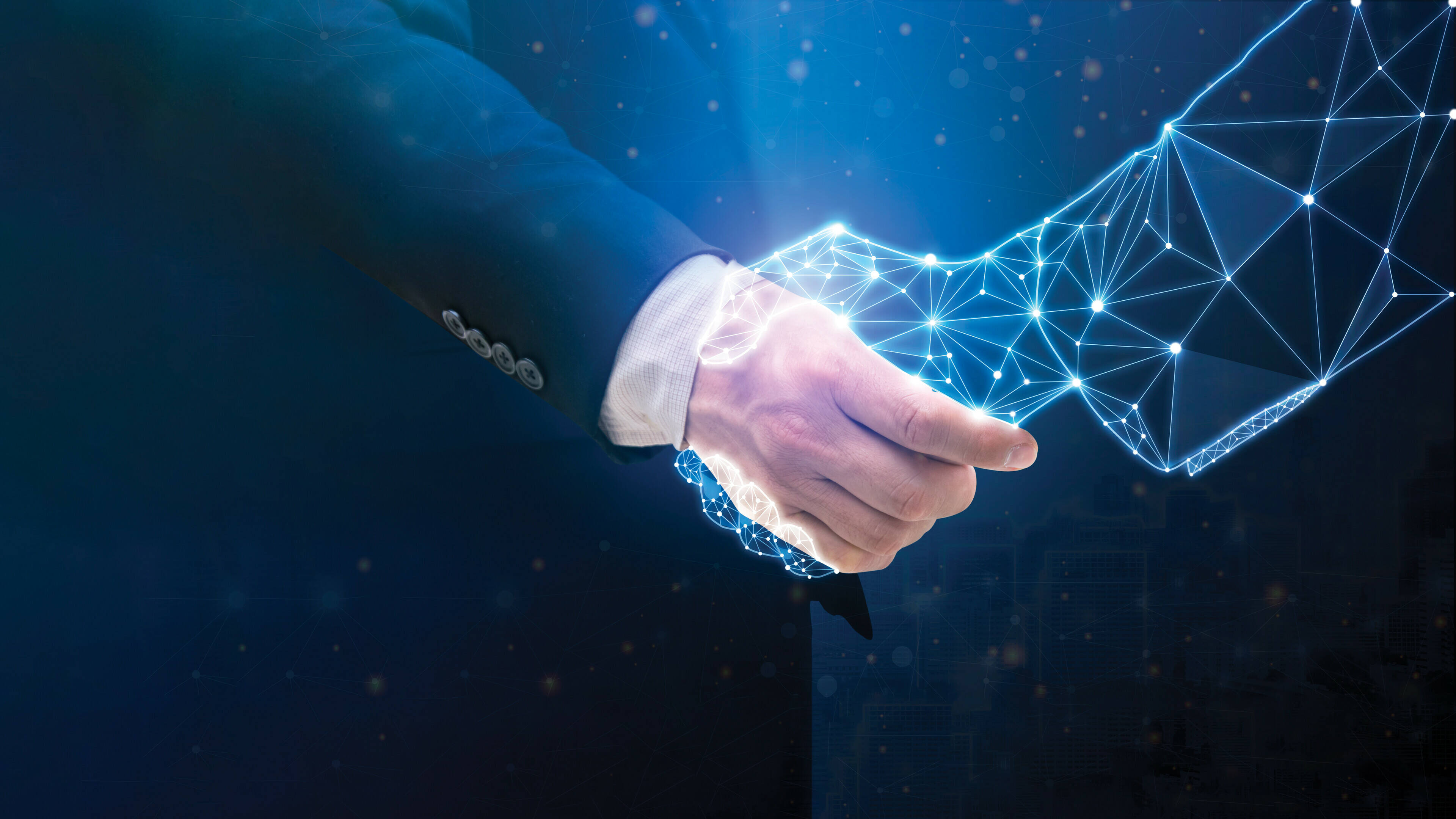
If you’re not convinced by AI yet, try this:
Airspace asked ChatGPT 4:
Artificial Intelligence (AI) is a broader concept that refers to machines or systems that can perform tasks that typically require human intelligence. These tasks include things like understanding natural language, recognising images, solving complex problems, and learning from experience. AI aims to create systems that can perform tasks intelligently and independently. AI includes a wide range of techniques and technologies, including machine learning, but also encompasses other methods like rule-based systems, expert systems, and more.
Machine Learning (ML) is a subset of AI and focuses specifically on the idea that machines can learn from data, identify patterns, and make decisions with minimal human intervention. It is centred on the development of algorithms that can learn from and make predictions or decisions based on data. ML systems improve their performance as they are exposed to more data over time. Techniques in machine learning include supervised learning, unsupervised learning, and reinforcement learning, among others.
With thanks to Prof. Gokhan Inalhan at Cranfield University
“What is artificial intelligence and machine learning and how do they differ?”
Trustworthy AI
AI will doubtless have an impact on ATM and force significant changes in ANSP business models. In the short-term, development will focus on tools that analyse and predict ATM operations. These will allow ANSPs to automate or get assistance in tactical decisions and evolve to include all stakeholders within the global ATM network, including operators, ANSPs, airports and manufacturers. Longer term, generative AI – a term for devising original content – will transform airspace design and associated procedures.
But there is a long way to go before the prediction of AI’s transformative role in ATM becomes a reality.
CANSO’s Artificial Intelligence whitepaper concludes: “Trustworthy AI has to begin by using sound engineering practices, mandated by agreed and accepted standards. Too much of AI in the commercial world thus far has consisted of short-term solutions, algorithms that get a system to work immediately, without the critical layer of safety assurance and engineering guarantees that are taken for granted within the aviation world.”
Certifying systems
Aside from correctly applying the human-machine interface, the main concern about AI in ATM is obtaining the necessary certification. Prof. Inalhan notes that “the underlying provision of verification and validation in support of certification” will be a complex undertaking.
Previously, the development lifecycle of a system meant that safety could be assured at every stage. The programming of a system was effectively transparent. AI, and in particular machine learning, a subset of AI, is a different concept entirely, as the algorithms are fully embedded within the data, making the process far more opaque.
AI’s ability to learn from experience therefore represents a new paradigm for software assurance, which goes from certifiable algorithms or coding to certifiable datasets and training programmes.
Safety-critical AI applications may also have automation as the ultimate goal. But as AI’s output cannot be fully explained from the outset, making an effective safety case may prove difficult.
Support for the AI certification process is not yet widespread though work is ongoing to produce the standards and guidance that will allow AI to be implemented with confidence.
This work includes:
European Aviation Safety Agency’s Artificial Intelligence Roadmap, which will be amended annually according to developments. The finalisation of EASA AI/ML policy is not expected until 2028.
International Air Transport Association’s (IATA) AI in Aviation that highlights some of the threats and opportunities in AI.
Various initiatives at ICAO.
The European Union Agency for Cybersecurity’s Artificial Intelligence Cybersecurity Challenges, which is also a crucial area for the industry to address.
A broad range of AI applications under the umbrella of the ISO/IEC JTC1/SC42, a reference for all parties developing AI-related standards.
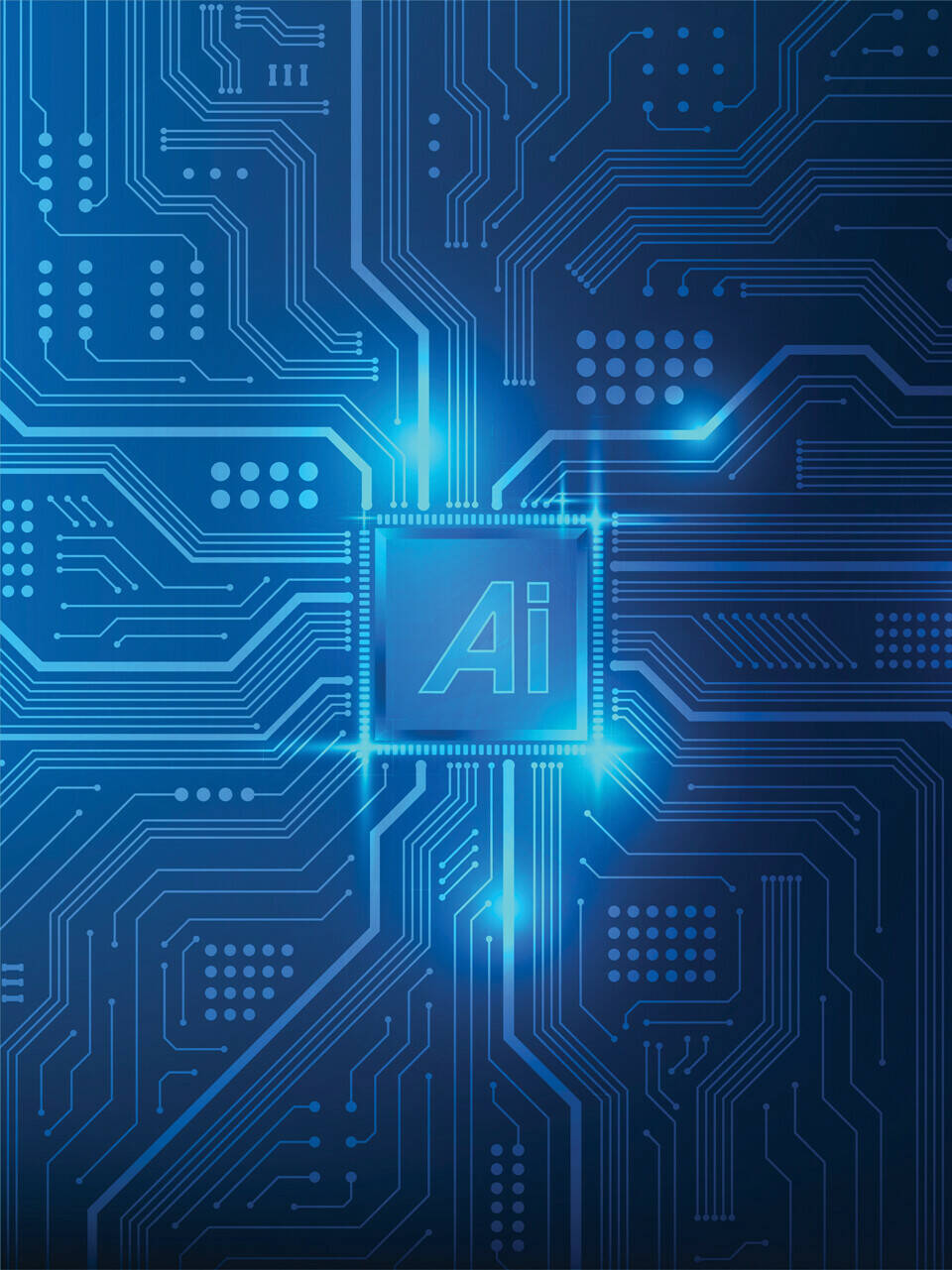
In all the above areas, AI will be the basis of increasing automation in the industry. Experts suggest Airborne Collision Avoidance Systems (ACAS), for example, will almost certainly be a fully automated function.
In general, however, an ATCO will always be involved and always have the final decision. Getting the balance right in this human-machine interface will be critical.
For most systems, AI will propose a solution that the ATCO can either accept or reject. This process creates a positive loop as human feedback and decision-making will allow the AI to learn, thus improving system resilience.
For example, AI may detect a problem, but it is up to the ATCO to assess that problem from an operational perspective. An ATCO may deem it necessary to override the AI in a given context while still maintaining an acceptable level of safety. This will often happen in the terminal area where domain expertise can best balance safety and efficiency.
Effectively, AI will do the bulk of the work, analysing, classifying and ordering flights as necessary. Anything out of the ordinary gets passed on to the human, who can then apply expertise and contextual information to resolve the issue.
Prof. Inalhan says human performance aspects, such as situational awareness, trust in the system, responsibilities and team composition are all vital factors in an AI-managed environment. He suggests the main problem will be harmonising advances across a complex, interconnected system that contains AI and humans in various roles.
The role of the human
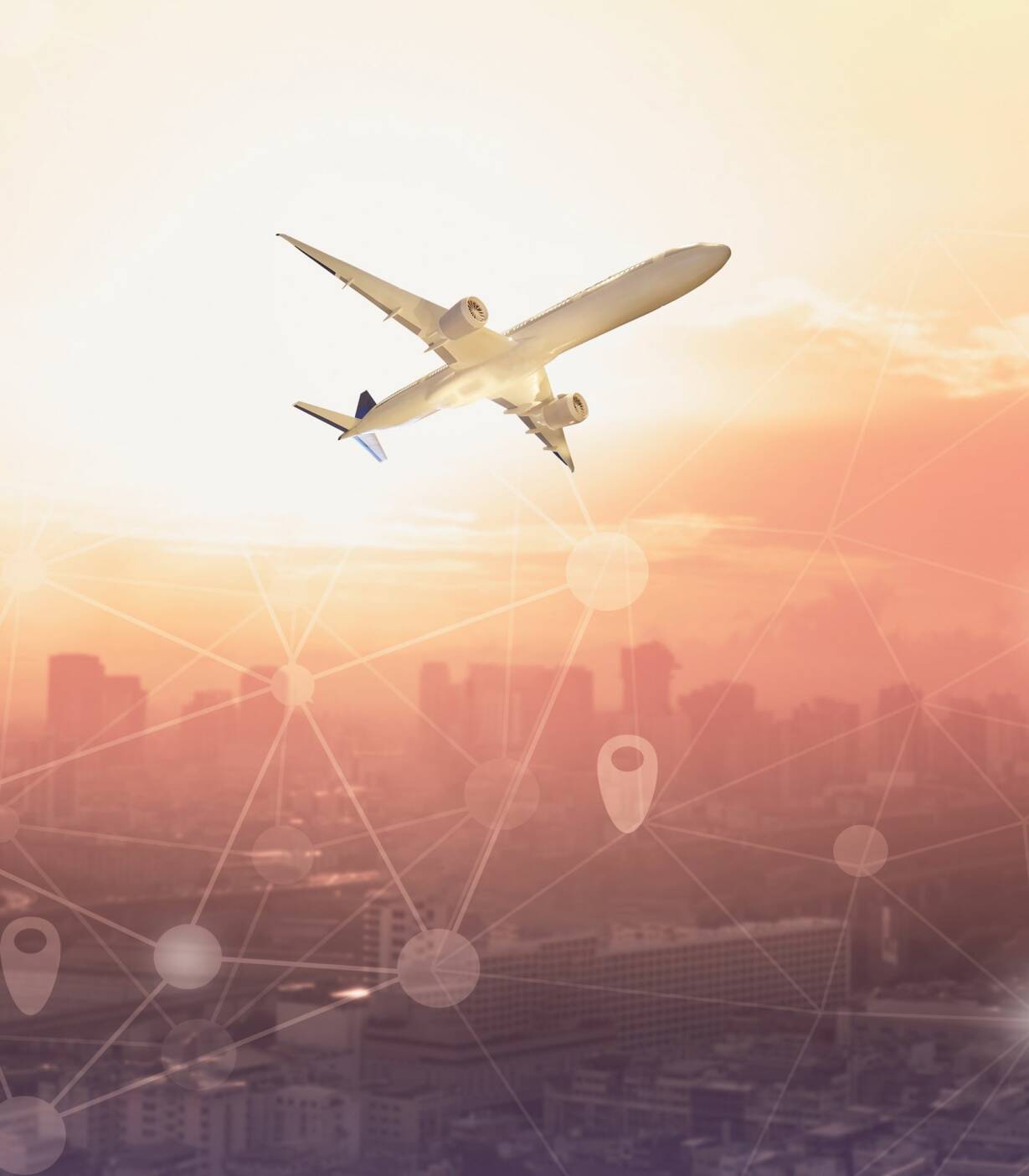
Where exactly will AI help ATM?
Conflict Resolution Advisory:
AI will help ATCOs detect and resolve conflicts in an optimised manner, such as ensuring preferred safety distance and reducing fuel consumption or delays.
Voice Recognition:
AI algorithms and methods can be used in various ways. The automation of communication between air traffic controllers and pilots will enable air traffic controllers to focus more on safety-critical, complex situations.
Optimal Sectorisation:
AI will improve dynamic airspace management. Traffic data and sector configuration information will enable AI to re-shape sector boundaries to better accommodate traffic.
Demand Prediction:
AI will improve the accuracy and predictability of traffic demand, making future planning more efficient
Remote Towers:
AI will play a vital role in pattern recognition, combining multiple camera angles and sifting through the multitude of data.
Unmanned Traffic Management:
AI will be crucial to ensuring safety as unmanned aerial vehicle traffic management (UTM) takes hold. It will provide solutions for conflict detection, traffic advisory and resolution, and cybersecurity. It will also facilitate increased levels of automation.
Predictive Maintenance:
AI will be able to detect unwanted behaviour and hidden failure patterns and alert engineers as necessary.
Weather Impact Prediction:
AI will not only help in weather prediction but also analyse weather’s impact on specific network resources like runways, sectors, airways, waypoints and airports.
“Too much of AI in the commercial world thus far has consisted of short-term solutions, algorithms that get a system to work immediately, without the critical layer of safety assurance and engineering guarantees that are taken for granted within the aviation world.”
CANSO’s Artificial Intelligence whitepaper
AI in ATM
But determining exactly what AI will do is not easy. It is a complex and nuanced area and the interaction with ATM only serves to compound the complications. AI will have to be integrated in an ATM ecosystem that itself is rapidly changing due to new airspace users, revamped procedures and evolving business models.
AI refers to systems that can perform tasks that would otherwise require human intelligence. Its main role will be dealing with the huge amounts of data that aviation produces daily. Computing power and advanced algorithms have combined with this data abundance to push AI to the fore.
Prof. Gokhan Inalhan, BAE Systems Chair, Professor of Autonomous Systems and Artificial Intelligence at Cranfield University, says the development will enable air navigation service providers (ANSPs) to make future demand-capacity projections, quantify risks and detect anomalies beyond existing levels of detail and accuracy.
“AI-based systems can provide decision support, aiding human cognition and decision-making, by enhancing situational awareness and providing suggestions and solutions starting at air traffic control officer (ATCO) level and going all the way up to ATM network management level,” he says. “This opens the door for automating almost any of the ATM tasks and decision-making, paving the way for far more efficient and safe ATM operations.”
It has become a well-known mantra: artificial intelligence (AI) is set to transform air traffic management (ATM).
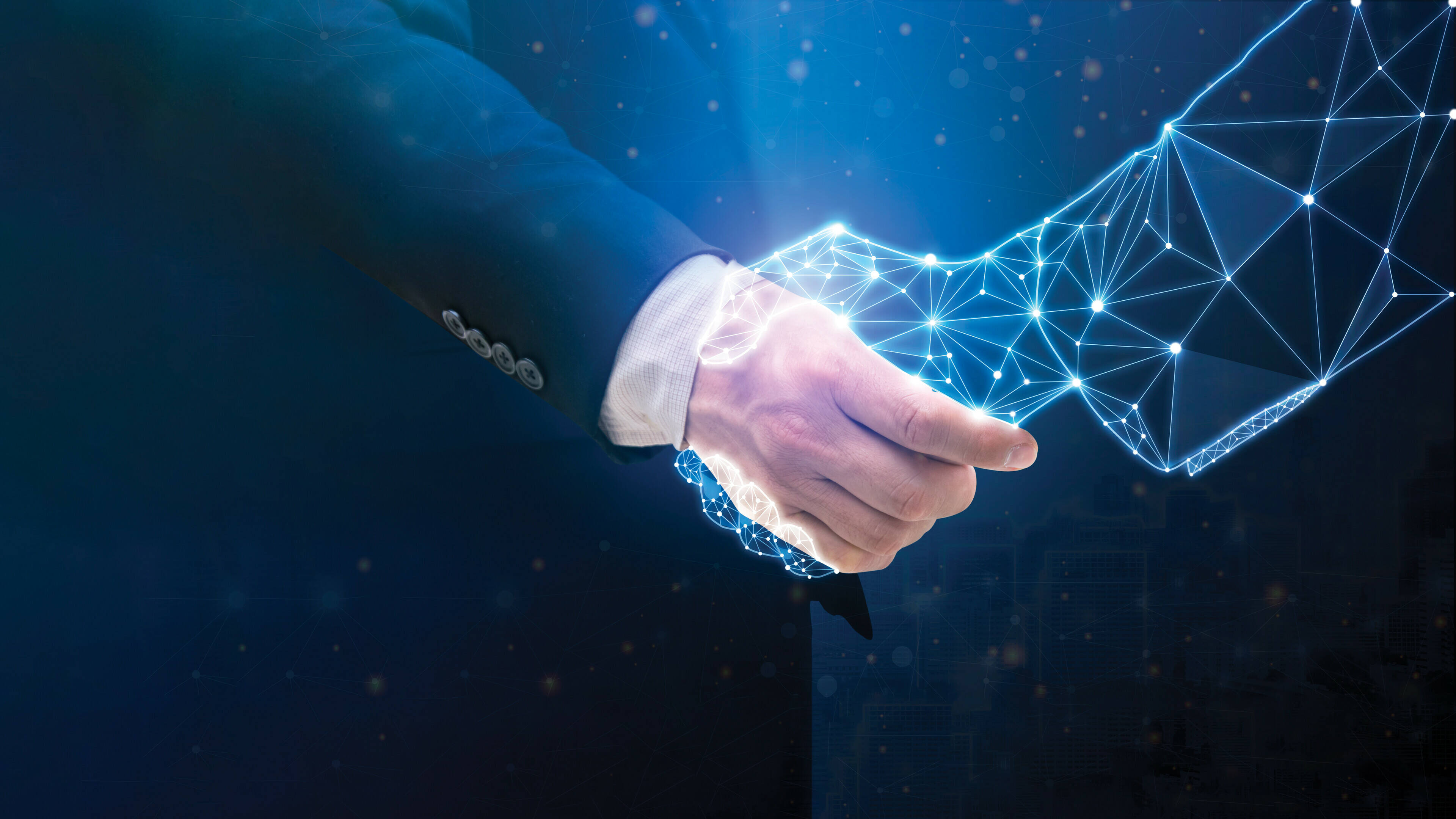
Artificial Intelligence (AI) is a broader concept that refers to machines or systems that can perform tasks that typically require human intelligence. These tasks include things like understanding natural language, recognising images, solving complex problems, and learning from experience. AI aims to create systems that can perform tasks intelligently and independently. AI includes a wide range of techniques and technologies, including machine learning, but also encompasses other methods like rule-based systems, expert systems, and more.
Machine Learning (ML) is a subset of AI and focuses specifically on the idea that machines can learn from data, identify patterns, and make decisions with minimal human intervention. It is centred on the development of algorithms that can learn from and make predictions or decisions based on data. ML systems improve their performance as they are exposed to more data over time. Techniques in machine learning include supervised learning, unsupervised learning, and reinforcement learning, among others.
With thanks to Prof. Gokhan Inalhan at Cranfield University
“What is artificial intelligence and machine learning and how do they differ?”
If you’re not convinced by AI yet, try this:
Airspace asked ChatGPT 4:
AI will doubtless have an impact on ATM and force significant changes in ANSP business models. In the short-term, development will focus on tools that analyse and predict ATM operations. These will allow ANSPs to automate or get assistance in tactical decisions and evolve to include all stakeholders within the global ATM network, including operators, ANSPs, airports and manufacturers. Longer term, generative AI – a term for devising original content – will transform airspace design and associated procedures.
But there is a long way to go before the prediction of AI’s transformative role in ATM becomes a reality.
CANSO’s Artificial Intelligence whitepaper concludes: “Trustworthy AI has to begin by using sound engineering practices, mandated by agreed and accepted standards. Too much of AI in the commercial world thus far has consisted of short-term solutions, algorithms that get a system to work immediately, without the critical layer of safety assurance and engineering guarantees that are taken for granted within the aviation world.”
Trustworthy AI
Aside from correctly applying the human-machine interface, the main concern about AI in ATM is obtaining the necessary certification. Prof. Inalhan notes that “the underlying provision of verification and validation in support of certification” will be a complex undertaking.
Previously, the development lifecycle of a system meant that safety could be assured at every stage. The programming of a system was effectively transparent. AI, and in particular machine learning, a subset of AI, is a different concept entirely, as the algorithms are fully embedded within the data, making the process far more opaque.
AI’s ability to learn from experience therefore represents a new paradigm for software assurance, which goes from certifiable algorithms or coding to certifiable datasets and training programmes.
Safety-critical AI applications may also have automation as the ultimate goal. But as AI’s output cannot be fully explained from the outset, making an effective safety case may prove difficult.
Support for the AI certification process is not yet widespread though work is ongoing to produce the standards and guidance that will allow AI to be implemented with confidence.
This work includes:
European Aviation Safety Agency’s Artificial Intelligence Roadmap, which will be amended annually according to developments. The finalisation of EASA AI/ML policy is not expected until 2028.
International Air Transport Association’s (IATA) AI in Aviation that highlights some of the threats and opportunities in AI.
Various initiatives at ICAO.
The European Union Agency for Cybersecurity’s Artificial Intelligence Cybersecurity Challenges, which is also a crucial area for the industry to address.
A broad range of AI applications under the umbrella of the ISO/IEC JTC1/SC42, a reference for all parties developing AI-related standards.
Certifying systems
In all the above areas, AI will be the basis of increasing automation in the industry. Experts suggest Airborne Collision Avoidance Systems (ACAS), for example, will almost certainly be a fully automated function.
In general, however, an ATCO will always be involved and always have the final decision. Getting the balance right in this human-machine interface will be critical.
For most systems, AI will propose a solution that the ATCO can either accept or reject. This process creates a positive loop as human feedback and decision-making will allow the AI to learn, thus improving system resilience.
For example, AI may detect a problem, but it is up to the ATCO to assess that problem from an operational perspective. An ATCO may deem it necessary to override the AI in a given context while still maintaining an acceptable level of safety. This will often happen in the terminal area where domain expertise can best balance safety and efficiency.
Effectively, AI will do the bulk of the work, analysing, classifying and ordering flights as necessary. Anything out of the ordinary gets passed on to the human, who can then apply expertise and contextual information to resolve the issue.
Prof. Inalhan says human performance aspects, such as situational awareness, trust in the system, responsibilities and team composition are all vital factors in an AI-managed environment. He suggests the main problem will be harmonising advances across a complex, interconnected system that contains AI and humans in various roles.
The role of the human
Conflict Resolution Advisory:
AI will help ATCOs detect and resolve conflicts in an optimised manner, such as ensuring preferred safety distance and reducing fuel consumption or delays.
Voice Recognition:
AI algorithms and methods can be used in various ways. The automation of communication between air traffic controllers and pilots will enable air traffic controllers to focus more on safety-critical, complex situations.
Optimal Sectorisation:
AI will improve dynamic airspace management. Traffic data and sector configuration information will enable AI to re-shape sector boundaries to better accommodate traffic.
Demand Prediction:
AI will improve the accuracy and predictability of traffic demand, making future planning more efficient
Remote Towers:
AI will play a vital role in pattern recognition, combining multiple camera angles and sifting through the multitude of data.
Unmanned Traffic Management:
AI will be crucial to ensuring safety as unmanned aerial vehicle traffic management (UTM) takes hold. It will provide solutions for conflict detection, traffic advisory and resolution, and cybersecurity. It will also facilitate increased levels of automation.
Predictive Maintenance:
AI will be able to detect unwanted behaviour and hidden failure patterns and alert engineers as necessary.
Weather Impact Prediction:
AI will not only help in weather prediction but also analyse weather’s impact on specific network resources like runways, sectors, airways, waypoints and airports.
Where exactly will AI help ATM?
AI refers to systems that can perform tasks that would otherwise require human intelligence. Its main role will be dealing with the huge amounts of data that aviation produces daily. Computing power and advanced algorithms have combined with this data abundance to push AI to the fore.
Prof. Gokhan Inalhan, BAE Systems Chair, Professor of Autonomous Systems and Artificial Intelligence at Cranfield University, says the development will enable air navigation service providers (ANSPs) to make future demand-capacity projections, quantify risks and detect anomalies beyond existing levels of detail and accuracy.
“AI-based systems can provide decision support, aiding human cognition and decision-making, by enhancing situational awareness and providing suggestions and solutions starting at air traffic control officer (ATCO) level and going all the way up to ATM network management level,” he says. “This opens the door for automating almost any of the ATM tasks and decision-making, paving the way for far more efficient and safe ATM operations.”
AI in ATM
But determining exactly what AI will do is not easy. It is a complex and nuanced area and the interaction with ATM only serves to compound the complications. AI will have to be integrated in an ATM ecosystem that itself is rapidly changing due to new airspace users, revamped procedures and evolving business models.
It has become a well-known mantra: artificial intelligence (AI) is set to transform air traffic management (ATM).